LG Innotek develops AI for raw material inspection
By applying AI to high-value semiconductor substrates, the company aims to reduce defect cause analysis time by up to 90%
By Sep 25, 2024 (Gmt+09:00)
When in S. Korea, it’s a ritual: Foreigners make stops at CU, GS25, 7-Eleven
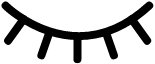
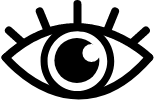
Maybe Happy Ending: A robot love story that rewrote Broadway playbook
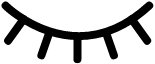
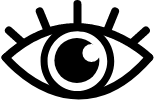
NPS yet to schedule external manager selection; PE firms’ fundraising woes deepen
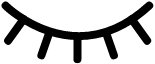
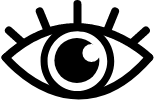
Samsung steps up AR race with advanced microdisplay for smart glasses
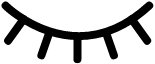
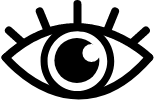
Seoul appeal: Korean art captivates Indonesia’s affluent connoisseurs
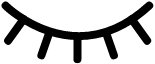
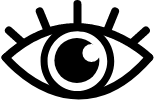
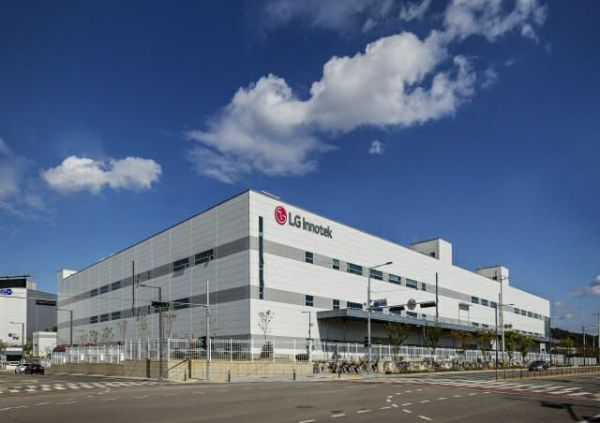
LG Innotek, a leading South Korean electronics parts maker, said on Wednesday it developed an artificial intelligence (AI) system that can filter out defective raw materials before they are used in production, significantly reducing the time and cost of quality control.
This AI system, which combines material information technology with AI image processing, was first introduced into the radio frequency system in package (RF-SiP) process and has recently been expanded to high-value semiconductor substrates, such as flip-chip ball grid arrays (FC-BGA).
To overcome the limitations of manual visual inspections, LG Innotek’s AI solution has been trained on tens of thousands of data samples to visualize the material compositions that are either acceptable or defective.
The system can analyze the composition of raw semiconductor materials and pinpoint defect areas within just one minute, achieving over 90% accuracy.
It also visualizes quality deviations by lot (a batch of raw materials with identical characteristics), allowing for more precise oversight.
Through machine learning, the AI optimizes the composition of materials to standardize visual and quantitative assessments, preventing defective raw materials from entering the production process.
LG Innotek said the AI implementation has reduced the time spent analyzing defect causes by up to 90%, leading to significant cost savings.
The company also plans to extend the application of this AI technology to its optical solutions product line, such as camera modules, where it can detect material defects using image-based analysis.
Write to Hyung-Kyu Kim at khk@hankyung.com
-
AutomobilesLG Innotek ups automotive electronics patents for future mobility
Aug 28, 2024 (Gmt+09:00)
2 Min read -
Tech, Media & TelecomLG Innotek, Ansys forge digital twin technology partnership
Aug 08, 2024 (Gmt+09:00)
1 Min read -
AutomobilesLG Innotek to foster car communication parts business with digital key
Jul 22, 2024 (Gmt+09:00)
2 Min read -
Tech, Media & TelecomLG Innotek begins supplying AI chip substrates to Big Tech
Jun 12, 2024 (Gmt+09:00)
3 Min read